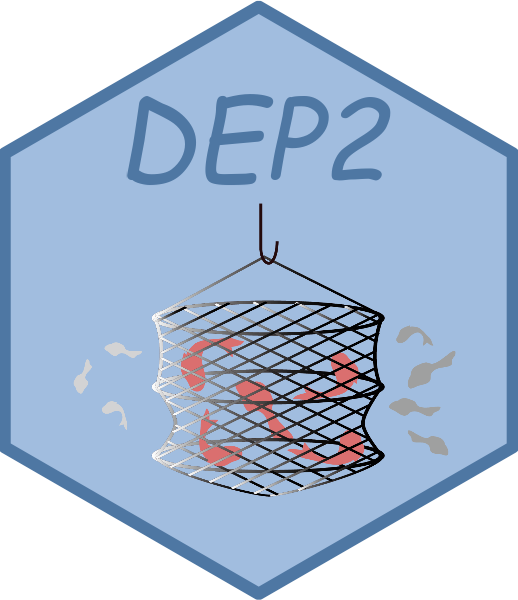
Extract significant candidates from SummarizedExperiment or DEGdata
get_signicant.Rd
Extract significant candidates from SummarizedExperiment or DEGdata
Usage
get_signicant(
object,
contrasts = NULL,
thresholdmethod = NULL,
diff = diff,
alpha = alpha,
curvature = 1,
x0_fold = 2,
return_type = c("subset", "table", "names")
)
Arguments
- object
a SummarizedExperiment or DEGdata object.
- contrasts
NULL or contrasts in object.
- thresholdmethod
NULL or 'intersect' or 'curve'. The thresholdmethod to decide significant. If is NULL, used existing rejections. Otherwise filter new rejections via
add_rejections
().- alpha
Numeric(1), Sets the threshold for the adjusted P value.
- curvature
Numeric(1), Sets the curvature for the curve cutoff lines
- x0_fold
Numeric(1), decide the x0 ratio to the standard deviations of L2FC. The x0 usually is set to 1(medium confidence) or 2(high confidence) standard deviations.
- return_type
One of "subset", "table", "names". "subset" return a subset object, "table" return a result data.frame, names return name vector of object.
Value
A object in the same class of input or a data.frame or a names vector, designed by return_type.
Examples
# Load example
data(Silicosis_pg)
data <- Silicosis_pg
data_unique <- make_unique(data, "Gene.names", "Protein.IDs", delim = ";")
# Differential test
ecols <- grep("LFQ.", colnames(data_unique))
se <- make_se_parse(data_unique, ecols,mode = "delim")
filt <- filter_se(se, thr = 0, fraction = 0.4, filter_formula = ~ Reverse != "+" & Potential.contaminant!="+")
#> filter base on missing number is <= 0 in at least one condition.
#> filter base on missing number fraction < 0.4 in each row
#> filter base on giving formula
norm <- normalize_vsn(filt)
#> vsn2: 8762 x 20 matrix (1 stratum).
#> Please use 'meanSdPlot' to verify the fit.
imputed <- impute(norm, fun = "MinProb", q = 0.05)
#> Imputing along margin 2 (samples/columns).
#> [1] 0.3026531
diff <- test_diff(imputed, type = "control", control = c("PBS"), fdr.type = "Storey's qvalue")
#> Tested contrasts: W10_vs_PBS, W2_vs_PBS, W4_vs_PBS, W6_vs_PBS, W9_vs_PBS
#> Storey's qvalue
dep <- add_rejections(diff, alpha = 0.01,lfc = 2)
# Signicant subset
(sig <- get_signicant(dep))
#> class: SummarizedExperiment
#> dim: 234 20
#> metadata(0):
#> assays(1): ''
#> rownames(234): Acp5 Adamts8 ... Ywhab Zbp1
#> rowData names(75): name Protein.IDs ... W9_vs_PBS_significant
#> significant
#> colnames(20): PBS_1 PBS_2 ... W9_2 W9_4
#> colData names(4): label ID condition replicate
# Given threshold
(sig <- get_signicant(dep, contrast = "W4_vs_PBS", alpha = 0.001, diff = 2))
#> class: SummarizedExperiment
#> dim: 70 20
#> metadata(0):
#> assays(1): ''
#> rownames(70): Adamts8 Add2 ... Treml1 Zbp1
#> rowData names(75): name Protein.IDs ... W9_vs_PBS_significant
#> significant
#> colnames(20): PBS_1 PBS_2 ... W9_2 W9_4
#> colData names(4): label ID condition replicate
# In table format
sig_df <- get_signicant(dep, return_type = "table")
head(sig_df)
#> name ID W10_vs_PBS_p.val W2_vs_PBS_p.val W4_vs_PBS_p.val
#> 1 Acp5 Q05117 1.411879e-05 5.910853e-04 4.778749e-03
#> 2 Adamts8 P57110 8.468615e-02 1.088907e-02 2.930611e-03
#> 3 Add2 Q9QYB8 2.273146e-04 5.390183e-03 1.325563e-04
#> 4 Aif1 O70200 9.869643e-06 1.275223e-07 2.825726e-07
#> 5 Alox12 P39655 3.538216e-03 3.931431e-03 4.797651e-03
#> 6 Ank1 Q02357 2.101051e-04 4.827090e-04 1.665043e-05
#> W6_vs_PBS_p.val W9_vs_PBS_p.val W10_vs_PBS_p.adj W2_vs_PBS_p.adj
#> 1 2.099924e-04 0.0003195929 0.000685 2.48e-03
#> 2 1.258278e-04 0.0023387008 0.094700 1.79e-02
#> 3 5.529725e-04 0.0072071771 0.002900 1.10e-02
#> 4 1.105552e-05 0.0007297945 0.000548 1.33e-05
#> 5 1.476942e-04 0.0054096376 0.012800 8.82e-03
#> 6 7.312216e-05 0.0000795242 0.002770 2.15e-03
#> W4_vs_PBS_p.adj W6_vs_PBS_p.adj W9_vs_PBS_p.adj W10_vs_PBS_significant
#> 1 1.33e-02 0.001180 0.00281 TRUE
#> 2 9.66e-03 0.000850 0.00878 FALSE
#> 3 1.55e-03 0.002060 0.01780 TRUE
#> 4 4.28e-05 0.000206 0.00446 TRUE
#> 5 1.33e-02 0.000936 0.01460 FALSE
#> 6 4.88e-04 0.000617 0.00129 TRUE
#> W2_vs_PBS_significant W4_vs_PBS_significant W6_vs_PBS_significant
#> 1 FALSE FALSE FALSE
#> 2 FALSE TRUE TRUE
#> 3 FALSE TRUE FALSE
#> 4 TRUE TRUE TRUE
#> 5 FALSE FALSE TRUE
#> 6 FALSE TRUE TRUE
#> W9_vs_PBS_significant significant W10_vs_PBS_ratio W2_vs_PBS_ratio
#> 1 FALSE TRUE 2.26 1.29
#> 2 TRUE TRUE 1.48 1.89
#> 3 FALSE TRUE -2.62 -1.46
#> 4 FALSE TRUE 2.56 2.92
#> 5 FALSE TRUE -1.80 -1.45
#> 6 TRUE TRUE -2.35 -1.76
#> W4_vs_PBS_ratio W6_vs_PBS_ratio W9_vs_PBS_ratio PBS_centered W10_centered
#> 1 0.994 1.44 1.69 -1.14 1.120
#> 2 2.290 3.27 2.89 -1.93 -0.448
#> 3 -2.260 -1.95 -1.72 1.57 -1.050
#> 4 2.760 2.07 1.69 -1.98 0.583
#> 5 -1.410 -2.12 -1.70 1.35 -0.457
#> 6 -2.420 -2.12 -2.58 1.75 -0.593
#> W2_centered W4_centered W6_centered W9_centered
#> 1 0.15100 -0.1470 0.3000 0.549
#> 2 -0.03990 0.3660 1.3400 0.967
#> 3 0.10500 -0.6920 -0.3810 -0.147
#> 4 0.94900 0.7840 0.0933 -0.283
#> 5 -0.10500 -0.0643 -0.7730 -0.351
#> 6 -0.00249 -0.6710 -0.3700 -0.826