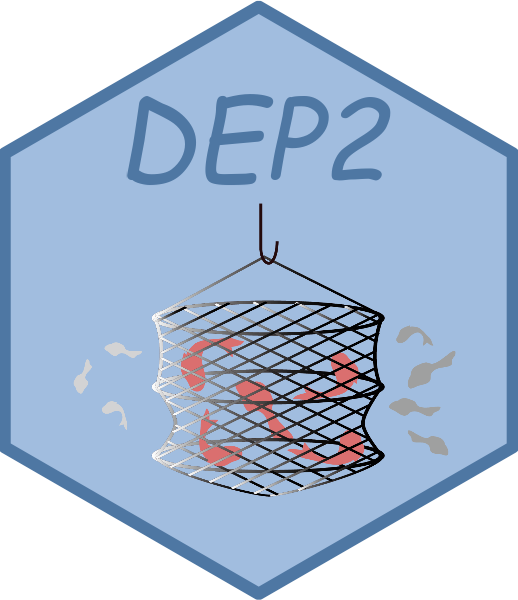
Plot PCA
plot_pca.Rd
plot_pca
generates a PCA plot using the top variable features
Usage
plot_pca(
object,
x = 1,
y = 2,
indicate = c("condition", "replicate"),
label = FALSE,
n = 500,
point_size = 4,
label_size = 3,
plot = TRUE,
features = "features",
if_square = FALSE
)
Arguments
- object
SummarizedExperiment (or DEGdata) object, Data object for which differentially enriched proteins are annotated (output from
test_diff()
(ortest_diff_deg()
) andadd_rejections()
).- x
Integer(1), Sets the principle component to plot on the x-axis.
- y
Integer(1), Sets the principle component to plot on the y-axis.
- indicate
Character, Sets the color, shape and facet_wrap of the plot based on columns from the experimental design (colData).
- label
Logical, Whether or not to add sample labels.
- n
Integer(1), Sets the number of top variable proteins to consider.
- point_size
Integer(1), Sets the size of the points.
- label_size
Integer(1), Sets the size of the labels.
- plot
Logical(1), If
TRUE
(default) the PCA plot is produced. Otherwise (ifFALSE
), the data which the PCA plot is based on are returned.- features
Character(1), the feature name in plot title, could be "proteins","genes", default is "features"
- if_square
Logical(1), if TRUE plot in a
Value
A scatter plot (generated by ggplot
).
Examples
# Load example
data(Silicosis_pg)
data <- Silicosis_pg
data_unique <- make_unique(data, "Gene.names", "Protein.IDs", delim = ";")
# Differential test
ecols <- grep("LFQ.", colnames(data_unique))
se <- make_se_parse(data_unique, ecols,mode = "delim")
filt <- filter_se(se, thr = 0, fraction = 0.4, filter_formula = ~ Reverse != "+" & Potential.contaminant!="+")
#> filter base on missing number is <= 0 in at least one condition.
#> filter base on missing number fraction < 0.4 in each row
#> filter base on giving formula
norm <- normalize_vsn(filt)
#> vsn2: 8762 x 20 matrix (1 stratum).
#> Please use 'meanSdPlot' to verify the fit.
imputed <- impute(norm, fun = "MinProb", q = 0.05)
#> Imputing along margin 2 (samples/columns).
#> [1] 0.3026531
# UMAP plot
plot_pca(imputed)
#> Warning: Use of `pca_df[[indicate[1]]]` is discouraged.
#> ℹ Use `.data[[indicate[1]]]` instead.
#> Warning: Use of `pca_df[[indicate[2]]]` is discouraged.
#> ℹ Use `.data[[indicate[2]]]` instead.